Harnessing the Power of Agriculture Dataset for Machine Learning
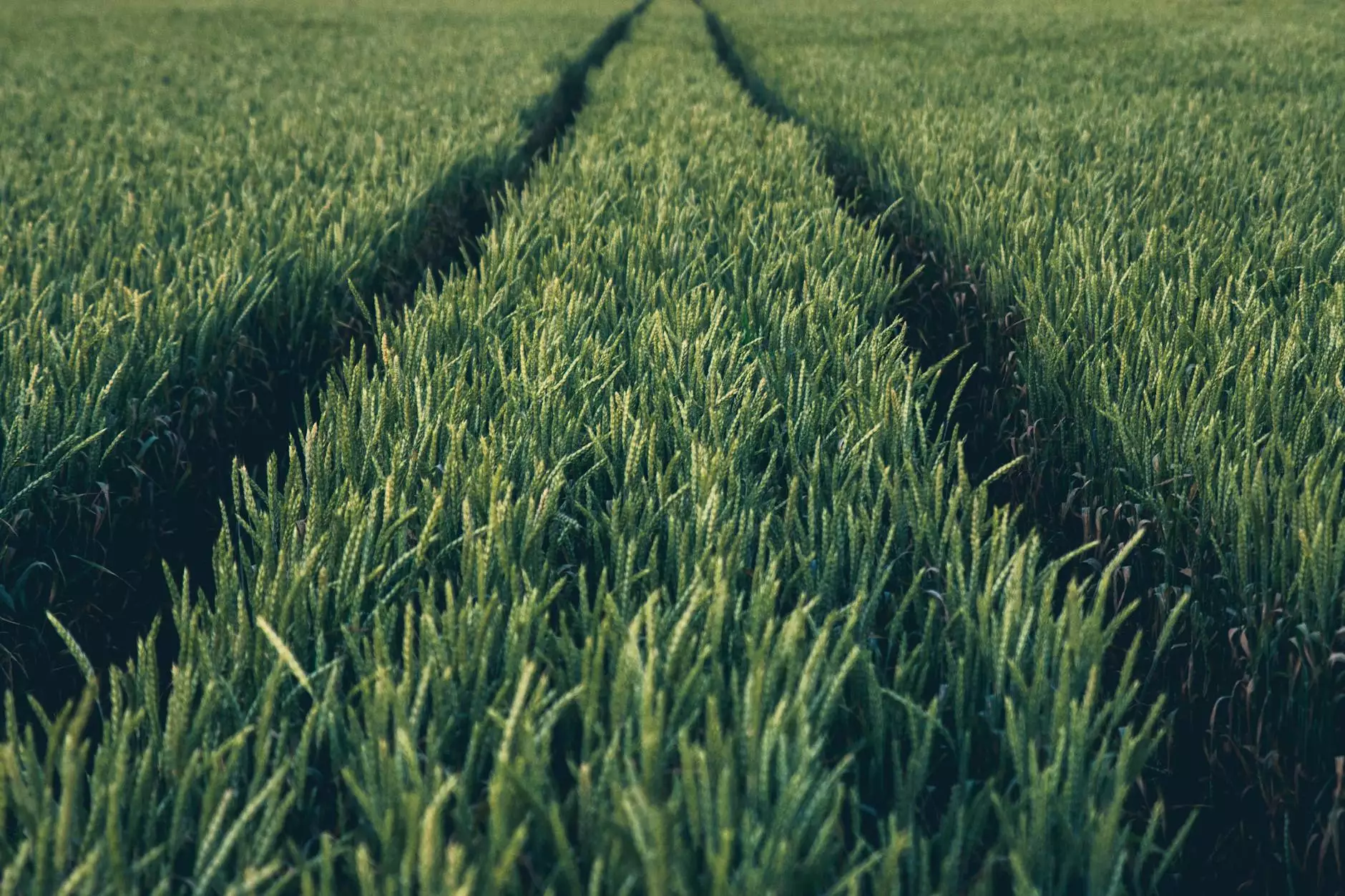
The Intersection of Agriculture and Technology
In the modern world, technology has become a crucial part of every industry. Agriculture, one of the oldest professions, is no exception. The integration of technology in farming not only enhances productivity but also ensures sustainability. One of the significant innovations in this area is the use of machine learning, particularly through the utilization of an agriculture dataset for machine learning.
What is an Agriculture Dataset for Machine Learning?
An agriculture dataset for machine learning comprises various types of data collected from agricultural practices. This data can include information about soil health, weather patterns, crop yields, and pest occurrences. By leveraging such datasets, farmers can make informed decisions that directly impact their productivity and efficiency.
- Soil Data: pH levels, nutrient content, moisture levels.
- Climate Data: temperature, precipitation, humidity levels.
- Crop Data: types of crops, yield statistics, growth rates.
- Pest and Disease Data: occurrences of pests, disease outbreaks, treatment effectiveness.
The Importance of Data in Modern Agriculture
The role of data in agriculture is undeniable. With the increase in global population and the corresponding demand for food, understanding agricultural practices through data analysis is essential. Here’s why:
- Enhanced Productivity: By analyzing past data, farmers can identify what practices yield the best results.
- Pest Management: Data can indicate when and where pests are likely to strike, helping farmers to act proactively.
- Resource Management: Understanding weather patterns assists in optimal resource allocation, such as water and fertilizers.
- Predictive Analysis: Machine learning models can predict future crop yields based on historical data.
Leveraging Machine Learning in Agriculture
Machine learning enables farmers to analyze vast amounts of data quickly and derive actionable insights. Here’s how:
1. Predictive Analytics
Using historical data, machine learning algorithms can predict future trends, such as expected crop yields under varying conditions. This allows farmers to prepare better and mitigate risks.
2. Disease Detection
Machine learning can be utilized to detect diseases in crops early by analyzing images taken from drones or sensors. By identifying symptoms before they become critical, farmers can take preventative measures.
3. Automated Systems
Automation in agriculture can also greatly benefit from machine learning. Automated watering and nutrient dispensing systems can adjust themselves based on the data analyzed from the agriculture dataset for machine learning.
Challenges in Implementing Machine Learning in Agriculture
Despite the advantages, there are several challenges in utilizing machine learning for agricultural purposes:
- Data Quality: The accuracy of the insights derived from machine learning models is directly dependent on the quality of the data collected.
- Cost of Implementation: Implementing advanced technological solutions can require significant investment, which may not be feasible for all farmers.
- Skill Gap: There is often a lack of understanding or skills to interpret machine learning outputs among farmers.
Case Studies of Successful Implementation
Numerous farmer cooperatives and agricultural businesses have successfully implemented machine learning initiatives that have significantly boosted their productivity:
Case Study 1: Predictive Analytics in Crop Management
A cooperative in the Midwest United States employed machine learning algorithms to analyze past climate data along with crop yield records. By identifying the conditions that led to optimal growth, they were able to increase their yield by an impressive 20% over three seasons.
Case Study 2: Smart Sensors for Real-time Monitoring
A farm in California deployed IoT devices that collected soil moisture levels and crop growth data. The data was processed using machine learning techniques to optimize irrigation schedules, leading to water savings of 30% while increasing crop yield.
The Future of Agriculture with Machine Learning
The future of agriculture looks promising with the continued integration of machine learning into everyday practices. Innovations such as:
- Blockchain for Data Sharing: Securely sharing agricultural data can improve accessibility and collaboration among farmers.
- Advanced Robotics: Using AI-driven robots can help in tasks such as planting, harvesting, and monitoring crops.
- Vertical Farming: Data-driven approaches can optimize urban agriculture practices, increasing food production in limited spaces.
Ultimately, the combination of these advancements creates a pathway to a more sustainable and productive agricultural landscape.
Conclusion
In conclusion, the utilization of an agriculture dataset for machine learning is revolutionizing the farming landscape. By empowering farmers with data-driven insights, we can increase efficiency, reduce costs, and promote sustainable practices. The intersection of agriculture and technology, specifically machine learning, is paving the way for a new era in agricultural productivity that meets the demands of our growing world.